Example: MRI Application
Contents
Example: MRI Application¶
In this example we take an image of the Shepp-Logan phantom and we evaluate the forward NUFFT on a set of points defining a radial k-space trajectory. Then, we use the the adjoint NUFFT to recover the image from the radial k-space data.
Prepare data¶
Let’s begin by creating an example image. We will use a Shepp-Logan phantom, generated using TensorFlow MRI.
import tensorflow as tf
import tensorflow_mri as tfmri
grid_shape = [256, 256]
image = tfmri.image.phantom(shape=grid_shape, dtype=tf.complex64)
print("image: \n - shape: {}\n - dtype: {}".format(image.shape, image.dtype))
image:
- shape: (256, 256)
- dtype: <dtype: 'complex64'>
Let us also create a k-space trajectory. In this example we will create a radial trajectory, also using TensorFlow MRI.
points = tfmri.sampling.radial_trajectory(base_resolution=256, views=233)
points = tf.reshape(points, [-1, 2])
print("points: \n - shape: {}\n - dtype: {}\n - range: [{}, {}]".format(
points.shape, points.dtype,
tf.math.reduce_min(points), tf.math.reduce_max(points)))
points:
- shape: (119296, 2)
- dtype: <dtype: 'float32'>
- range: [-3.1415927410125732, 3.141521453857422]
The trajectory should have shape [..., M, N]
, where M
is the number of
points and N
is the number of dimensions. Any additional dimensions ...
will
be treated as batch dimensions.
Batch dimensions for image
and traj
, if any, will be broadcasted.
Spatial frequencies should be provided in radians/voxel, ie, in the range
[-pi, pi]
.
Finally, we’ll also need density compensation weights for our set of nonuniform
points. These are necessary in the adjoint transform, to compensate for the fact
that the sampling density in a radial trajectory is not uniform. Here we use
tensorflow-mri
to calculate these weights.
weights = tfmri.sampling.radial_density(base_resolution=256, views=233)
weights = tf.reshape(weights, [-1])
print("weights: \n - shape: {}\n - dtype: {}".format(weights.shape, weights.dtype))
weights:
- shape: (119296,)
- dtype: <dtype: 'float32'>
Forward transform (image to k-space)¶
Next, let’s calculate the k-space coefficients for the given image and trajectory points (image to k-space transform).
import tensorflow_nufft as tfft
kspace = tfft.nufft(image, points,
transform_type='type_2',
fft_direction='forward')
print("kspace: \n - shape: {}\n - dtype: {}".format(kspace.shape, kspace.dtype))
kspace:
- shape: (119296,)
- dtype: <dtype: 'complex64'>
We are using a type-2 transform (uniform to nonuniform) and a forward FFT
(image domain to frequency domain). These are the default values for
transform_type
and fft_direction
, so providing them was not necessary in
this case.
Adjoint transform (k-space to image)¶
We will now perform the adjoint transform to recover the image given the
k-space data. In this case, we will use a type-1 transform (nonuniform to
uniform) and a backward FFT (frequency domain to image domain). Also note that,
prior to evaluating the NUFFT, we will compensate for the nonuniform sampling
density by simply dividing the k-space samples by the density weights.
Finally, for type-1 transforms we need to specify an additional grid_shape
argument, which should be the size of the image. If there are any batch
dimensions, grid_shape
should not include them.
# Apply density compensation.
comp_kspace = kspace / tf.cast(weights, tf.complex64)
recon = tfft.nufft(comp_kspace, points,
grid_shape=grid_shape,
transform_type='type_1',
fft_direction='backward')
print("recon: \n - shape: {}\n - dtype: {}".format(recon.shape, recon.dtype))
recon:
- shape: (256, 256)
- dtype: <dtype: 'complex64'>
Finally, let’s visualize the images.
import matplotlib.pyplot as plt
fig, ax = plt.subplots(1, 2)
ax[0].imshow(tf.abs(image), cmap='gray')
ax[0].set_title("Original image")
ax[1].imshow(tf.abs(recon), cmap='gray')
ax[1].set_title("Image after forward\nand adjoint NUFFT")
Text(0.5, 1.0, 'Image after forward\nand adjoint NUFFT')
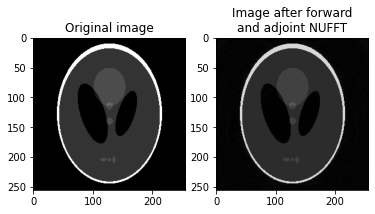